As advancements in AI technology progress, chatbots like ChatGPT, based on large language models, have become our preferred solutions for problem-solving. However, when posed with highly personalized, concrete, and contextual questions, their responses often fall short.
For instance, take this question, “I want to learn swimming. Can you give me some advice?” ChatGPT can only offer generic suggestions, such as “breathing techniques” or “how to float.” These answers, while not incorrect, fail to address the user’s specific situation and remain quite general. But imagine a coach friend accompanying you to the pool, demonstrating breath-holding techniques, supporting your waist for proper flotation, and guiding you on body control for buoyancy. Wouldn’t that be the kind of answer you’re really looking for?
This scenario underscores the value of ‘Embodied Agents.’ They emphasize the need to not only make computer programs intelligent but also enable them to interact closely with the real physical world, much like humans do. Only with these capabilities can we aspire to achieve an Artificial General Intelligence (AGI) that mirrors human intelligence and experience.
1. Why Must AI Embrace Embodied Intelligence?
Why do we seek artificial intelligence that interacts closely with the physical world and mirrors human intelligence? Isn’t it enough to regard AI as a convenient and practical tool?
This pursuit stems from a fundamental human expectation of intelligence: we hope that AI can not only efficiently perform complex tasks such as learning, problem-solving, and pattern recognition, aiding us in tasks we are unwilling or unable to undertake, but also understand human thought processes, behavioral habits, emotional expressions, and even personality preferences and psychological traits, achieving a deeper level of ‘understanding you.’ Furthermore, humans instinctively favor entities that feel more natural and relatable, while they often feel averse to purely mechanical, emotionless tools.
In 1950, Alan Turing first introduced the basic concept of artificial intelligence in his paper, along with the famous “Turing Test” to determine whether a machine could simulate human intelligence. In the same year, Isaac Asimov, in his short story collection “I, Robot,” depicted a future world where humans coexist with AI and proposed the three laws of robotics. Since the inception of the AI concept, humanity has believed in and yearned for an AI that can communicate in human language and understand us — not just as a companion in life but also bound by ethical constraints and ultimately guided by human emotions and personality.
Thus, when discussing ‘intelligence,’ we are essentially aspiring for AI to transcend mere computational machines, becoming entities with creative thinking and perceptive abilities, on par with human intelligence. Embodied intelligence represents the pathway to realizing this vision.
2. How Can Embodied Intelligence Resemble Humans?
So, how should embodied intelligence achieve a more human-like AI?
First, we need to understand the limitations of traditional artificial intelligence. Current AI systems primarily rely on internet-collected images, videos, or textual data for learning. Although well-crafted, these datasets are ultimately static and organized, labeled by humans. This limitation hinders AI’s ability to engage in meaningful dialogue and interaction with its environment. AI lacks the capacity to grasp the underlying logical pathways of its responses, much less engage in self-reflection and growth. Consequently, AI-generated data, apart from mimicking existing models, often misaligns with reality, leading to nonsensical outputs. This is a primary reason why traditional AI is termed ‘weak intelligence.’
In response, some scholars, grounded in studies of human infant cognition, draw inspiration from the development of human intelligence. They posit that true intelligence stems from continuous interaction and feedback with the surrounding environment. Just as human infants develop cognitive abilities through sensory perception and physical interaction with their environment, the real advancement of intelligence needs to surpass processing abstract information and deeply understand and respond to the complex situations of the real world. This understanding serves as the foundational premise for the concept of embodied intelligence.
Specifically, embodied intelligence is an intelligent system based on physical body perception and action. It acquires information, understands problems, makes decisions, and implements actions by interacting with the environment, thereby generating intelligent behavior and adaptability. As Professor Fei-Fei Li of Stanford University once pointed out, ‘Embodiment is not about the body itself, but rather about the overall need and function of interacting with the environment and engaging in activities within it.’ Similarly, Professor Cewu Lu of Shanghai Jiao Tong University vividly illustrates this through the analogy of a cat learning to walk, stating, ‘A freely moving cat epitomizes embodied intelligence as it autonomously navigates its environment, learning to walk. In contrast, a cat that merely passively observes the world may ultimately lose the ability to walk.’
Unlike traditional AI trained on static datasets, embodied intelligence learns and interacts in the real physical world in real-time, thus better simulating the human learning process. Much like humans, these systems gain knowledge and experience through direct interaction with the environment, comprehend real-time human feedback and behaviors, and grasp non-verbal communication methods, including perceiving and responding to human emotions through expressions and tactile feedback. This deep human-machine interaction and understanding make embodied intelligence a form of intelligence that is closer to human cognition and emotion, promising to achieve deeper levels of human-machine interaction and integration.
3. How Does Embodied Intelligence Achieve a More Human-like Presence?
Proactivity
As one of the core features of embodied intelligence, proactivity endows intelligent systems with the capacity to transcend passive information processing tools, transforming them into active participants.
In Metin Sitti’s 2021 paper ‘Physical Intelligence as a New Paradigm‘, he observes that in the realm of embodied physical intelligence, flexible systems not only respond to environmental stimuli … but also ascertain their positioning through self-localization of body parts, considering environmental conditions, self-movement, and proprioception. They then translate this awareness into subsequent actions. Similarly, the paper ‘Embodied Intelligence in Physical, Social and Technological Environments’ defines embodied intelligence as when a life form autonomously takes action in its environment, based on a variety of sensory information and, in doing so, distinguishes itself as a proactive, multi-sensory self, thereby modulating its interaction with ongoing events, it possesses embodied intelligence.
This proactivity can be understood through a simple analogy: when you walk into a library and meet a traditional librarian, they might respond to your request with a desired answer, like a book title and its location. However, if this librarian were an embodied intelligence guide, they would not only find the information you need but also actively lead you to the book, explain related knowledge, and immerse you in the world of that knowledge.
This interaction is like exploring knowledge with an enthusiastic, friendly companion, as opposed to merely receiving answers from an indifferent knowledge assistant. Through proactivity, embodied intelligence offers a novel interactive experience, enhancing human access to and understanding of information, and deepening the emotional and cognitive connection between humans and intelligent systems.
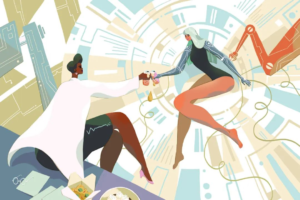
Author: Kim Salt
Although current embodied intelligence has not fully realized proactivity and enthusiastic interaction, the rapid development of visual navigation serves as an illuminating example. In challenges like iGibson Sim2Real, Habitat, and RoboTHOR, we have witnessed the emergence of this field’s preliminary forms, accomplishments that have transcended the indifference of mere task execution. For instance, navigation systems that integrate human prior knowledge, embedding it in deep reinforcement learning frameworks as multimodal inputs like knowledge graphs or audio, can enable AI to navigate unknown environments and discover previously unseen objects.
The cutting-edge Visual Language Navigation (VLN) technology aspires to create a form of embodied intelligence that can communicate with humans using natural language and autonomously navigate in real 3D environments. This field has already harnessed several datasets for research and development, such as REVERIE, R2R, CVDN, GELA, ALFRED, Talk2Nav, Touchdown, and has also produced some innovative network architectures like the auxiliary reasoning navigation framework. These technologies, applied in areas like machine navigation, assistive technology, and virtual assistants, are still in their nascent stages.
Furthermore, the advancement of VLN into visual dialogue navigation seeks to train AI in engaging in continuous natural language conversations with humans, thereby enhancing navigational assistance. In this domain, researchers have employed a Cross-Modal Memory Network (CMN), which possesses distinct language and visual memory modules, for remembering and understanding information related to past navigation actions, utilizing this data to make navigation decisions.
Real-Time Responsiveness
Real-time responsiveness is another core characteristic of embodied intelligence, enabling intelligent systems to learn promptly and respond swiftly in the real world. An embodied intelligent system with real-time capabilities can instantly react to new information or unfamiliar environments. In contrast, traditional artificial intelligence, reliant on pre-trained data, struggles to react quickly to real-time environmental changes.
In the case of TV shows, In the case of TV shows, watching a recorded magic show resembles interacting with traditional AI: the content may be captivating, but you are confined to passively viewing pre-recorded material, unable to interrupt or modify the content in real-time. Conversely, watching a live magic performance mirrors the experience of interacting with embodied intelligence: you can issue real-time requests, and the magician improvises on the spot, tailoring the act to your personal needs. You’re no longer just a passive viewer but an integral part of the magic show. This interaction is not only more personalized but also more engaging.
Thus, like a magician performing live, embodied intelligence can respond instantaneously to human needs and environmental changes, providing solutions that are more aligned with real-world scenarios and interacting with humans in a manner closer to interpersonal communication. This real-time responsiveness helps it integrate better into daily human life, becoming a more intelligent and useful companion, rather than just a machine executing predefined tasks.
The paper ‘LLM-Planner: Few-Shot Grounded Planning for Embodied Agents with Large Language Models’ introduces the LLM-Planner method. This approach leverages the capabilities of large language models for few-shot planning for embodied intelligence, enhancing the language models with a physical basis to generate and update plans relevant to the current environment. Its advantage lies in its ability to reflect and adapt to environmental changes in real time, providing immediate information and guidance for the decision-making of embodied intelligence.
Contextuality
Beyond proactivity and real-time response, embodied intelligence must possess profound perception and personalized understanding of specific scenes and contexts.
Like humans dynamically adjusting their behavior during real-time interactions with their surroundings, embodied intelligence should deeply understand its context through real-time learning and feedback, thereby adjusting its behavior accordingly. It should flexibly modify its responses based on contextual and environmental changes to integrate seamlessly into any given situation, facilitating more natural and effective communication. For instance, embodied intelligence could detect changes in a user’s mood and provide a personalized experience, enhancing user engagement and satisfaction.
Take travel planning as an example. Traditional chat intelligence might offer fixed itinerary suggestions regardless of weather conditions, perhaps even suggesting an outdoor hot spring visit during a thunderstorm. In contrast, embodied intelligence could offer more practical suggestions based on the user’s personal preferences, local environment, and weather conditions. It acts akin to a private travel consultant or a personal photographer, attuned to local nuances. It knows not just your destination but also the surrounding context and environmental changes. It can take you to the right small eatery based on your personal preferences and local seasonality, and capture every joyful moment.
Numerous realistic and publicly available 3D scenes exist as simulated environments for training embodied intelligence. For embodied navigation, there are virtual environments like iGibson, Habitat, MultiON, BEHAVIOR; for embodied question answering, there’s ALFRED; environments focusing on scene understanding, object states, and task planning include AI2-THOR, ThreeDWorld, Habitat 2.0; for object manipulation, there are SAPIEN, RLBench, VLMbench, RFUniverse, ARNOLD; datasets for object grasping and manipulation include GraspNet, SuctionNet, DexGraspNet, GAPartNet. These scenes, far more realistic than those in previous simulators, significantly advance the preliminary development of embodied intelligence in contextuality.
Furthermore, technological advancements in the sensing field provide reliable support for the development of contextual embodied intelligence. For example, the PaLM-E team proposed a concretized language model, integrating real-world continuous sensor modalities directly into the language model, thereby establishing a connection between words and perception. The inputs for this model are multimodal statements, intertwining visual, continuous state estimation, and text input encoding. Combining pre-trained large language models, these encodings are trained end-to-end for various specific tasks, such as sequential robotic operation planning, visual question answering, and image-video captioning, effectively building a link between words and perception.
Biomimicry
In comparison to general artificial intelligence, embodied intelligence is required to navigate complex environments and coexist with the real world in a manner closer to human cognition, which endows it with more biomimetic characteristics.
Much like a swarm of bees collaborates to construct a hive, multiple agents within embodied intelligence can work together to produce a collective effect that surpasses the capabilities of any single agent. This group collaboration not only exceeds the abilities of individual agents but also demonstrates the emergence phenomenon in complex systems. Within these systems, simple actions and interactions of individual agents can lead to complex behavior patterns and structures in the whole system, enabling it to adapt to new environments and tasks without reliance on pre-programmed rules.
Moreover, self-organization in embodied intelligence systems is a key aspect of their biomimetic nature. Agents can dynamically adjust their behavior and structure in response to environmental changes and interactions, forming higher-level functions and structures, thereby endowing the system with greater robustness and adaptability.
These characteristics of embodied intelligence have been exemplified in various applications. One research team designed an underwater soft robot inspired by the form of bacteria. This bio-inspired modular structure enables the robot to perform a variety of tasks in underwater environments. The robot utilizes its surrounding environment (water), the shape of the target, and its own compliance, achieving effective navigation and safe interaction with minimal control input. This modeling approach and design not only showcase the innovation of embodied intelligence in mimicking biological entities but also its multifunctionality and adaptability in practical applications.
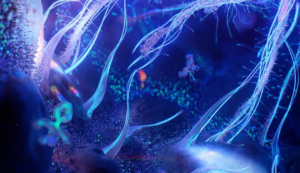
Author: Alexey Kashpersky & Newt Studios
In conclusion, the technological development in the field of embodied intelligence is trending towards diversification and integration, particularly in terms of advancements in observation, manipulation, and navigation. These developments are not just focused on a specific aspect of embodied intelligence but integrate various functions and capabilities to achieve higher adaptability and flexibility.
By integrating robots’ sensor data with general visual language data for joint training and utilizing the powerful intrinsic knowledge of large language models, embodied intelligence can effectively engage in dynamic learning and generalization in complex and unknown real-world environments. For instance, an LLM-based Agent (Large Language Model-based Agent) uses its unique linguistic abilities not only for environment interaction but also for transferring foundational skills to new tasks, thus enabling the robot to adapt to various operational environments through human language instructions.
Moreover, through embedded action planning, which utilizes high-level strategic guidance to define sub-goals for lower-level strategies and generate appropriate action signals, robots can execute tasks more efficiently and with greater control. For more effective navigation and other intricate tasks, embodied intelligence also requires memory buffers and summarization mechanisms to reference historical information and better adapt to unknown environments.
In recent years, Google’s Everyday Robot project’s SayCan system has successfully combined robots and dialogue models to complete a 16-step task; Berkeley’s LM Nav project has enabled a robot to reach destinations following verbal instructions, leveraging three large models (ViNG, GPT-3, and CLIP); and the PaLM-E model, a collaboration between Google and Technische Universität Berlin, has advanced multimodal understanding and execution within embodied intelligence.
It is evident that the technological development of embodied intelligence is moving towards a more integrated, flexible, and efficient direction. The fusion and advancement of these technologies not only enhance the adaptability and practicality of intelligent systems but also pave new pathways for future intelligent system design and application. With continuous technological progress, we can anticipate more practical applications and innovative breakthroughs in various fields of embodied intelligence.
4. The Relationship Between Artificial and Human Intelligence
To thoroughly understand the differences between Artificial Intelligence (AI) and Human Intelligence (HI) and explore ways to bridge this gap, the Shanda AI Lab LEAF team has proposed ‘Five Principles,’ considering the characteristics of embodied intelligence. These principles aim to analyze the development direction of AI and will be continuously expanded in the subsequent ‘Intelligence Asymptote’ series of reports. These principles not only echo the four major characteristics of embodied intelligence but also delve into the key aspects of AI development, with the hope of bringing AI closer to the complexity and adaptability of human intelligence.
Logicality
AI should possess logical thinking and understanding abilities akin to the human brain. Specifically, AI should be able to perform comprehensive calculations and reasoning within complex social scenarios, combining various stored knowledge, to understand semantics and the complex connotations behind them, ultimately producing corresponding outputs.
Perceptivity
AI needs robust perception capabilities to recognize and associate multiple signals and to engage in human-like imagination and synesthesia. It should understand chat inputs and process various types of information, reacting quickly to changes and stimuli in its surroundings, much like a human would.
Real-Time Responsiveness
AI systems should be capable of real-time information updating, retrieval, and environment-responsive feedback; they can learn from human memory modules, using context learning and situational learning, to analogously understand new tasks from limited real-time information.
Proactivity
AI should accomplish tasks similar to human functional processing through active, purposeful behavior, including setting goals, planning processes, breaking down tasks, employing tools and methods, managing time, and organizing arrangements. This means AI needs to glean from a wealth of real-world experiences, adjust in real-time to contexts and specific situations, and autonomously make decisions based on actual scenarios, thereby planning flexibly and interacting actively.
Adaptability
AI should actively perceive and understand the world, engaging in bidirectional, dynamic interactions with the environment. This adaptability is not limited to machine response to inputs but also includes the system’s ability to make appropriate decisions based on internal knowledge and alter its surroundings through specific actions. Sociologically, it means AI can interact with the world in a human-like manner and understand its complexity.
Evidently, to bring AI closer to human wisdom, it must understand and learn how humans cognitively perceive the world and then act in a manner akin to human decision-making.
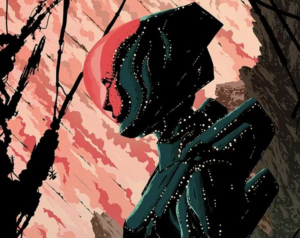
Author: Victorien Aubineau
Humans, as exemplars of strong intelligence, rely less on the supervised learning paradigm prevalent in deep learning during their growth. Instead, they develop key skills such as walking, using tools, and acquiring new abilities through hands-on trials. Similarly, embodied intelligence, though facing the instability of first-person perspective data in its interaction with the environment, can learn through a human-like central perception method and truly adapt and understand in real environments, thereby transitioning from vision, language, and reasoning to Artificial Embodiment.
5. The Development of Embodied Intelligence
Recently, ’embodied intelligence’ has emerged as a prominent research area, captivating fields like computer vision, natural language processing, and robotics. Since the inaugural Conference on Robot Learning (CoRL) in 2017, the field of robot learning has rapidly evolved, marked by the emergence of numerous new intelligent tasks, algorithms, and environments. Particularly during the CoRL conferences of 2018 and 2019, a wide array of academic tasks related to embodied intelligence, including embodied visual navigation and question answering systems, began garnering significant attention.
By 2023, the CVPR Embodied Intelligence Workshop organized challenges in AI Habitat, AI2-THOR, iGibson, and Sapien simulators, focusing on object rearrangement, embodied question answering, navigation, and robotic manipulation. These embodied intelligence tasks represent a completely different paradigm from other online AI tasks, involving embodied agents (such as robots) to “see,” “speak,” “listen,” “move,” and “reason” in order to interact with and explore their environment, thereby addressing various challenging tasks within it.
In summary, it is a growing trend for artificial intelligence to learn and understand the cognitive paradigms of the human brain and thereby come closer to human wisdom. Embodied intelligence, particularly the simulation of human-like embodied intelligence, represents a feasible and efficient pathway for artificial intelligence to approximate human intelligence.