The Brain: The Universe’s Most Complex System. For a long time, humans have endeavored to unravel the brain’s inner mechanisms, yet progress has been somewhat sluggish, constrained by technological and methodological limitations. However, with the advent of artificial intelligence technology, unprecedented opportunities have emerged for brain science research.
On June 21st, the Tianqiao and Chrissy Chen Institute (TCCI), in collaboration with Neurochat, initiated the fourth TCCI-Neurochat Online Academic Conference. On the opening day, Professor Xiao-Jing Wang from New York University delivered a keynote address titled ‘Theoretical Neuroscience in the Age of Artificial Intelligence’. He shared insights into the integration of artificial intelligence with neuroscience from a theoretical perspective, aiming to advance research in the field.
Theoretical or Computational Neuroscience involves applying mathematical theories to understand how the brain operates. Similar to the role of theoretical physics in the field of physics, theoretical neuroscience plays a crucial role in brain research, complementing experimental studies. Experimentation alone may not fully reveal how the brain functions on multiple levels. Theoretical neuroscience transforms neurons and neural networks into mathematical models and algorithms, thus building a bridge between brain science and AI. With advancements in experimental tools and the advent of big data, theoretical research and modeling have become increasingly essential.
Brain Functions Beyond AI’s Reach
The technological revolution in the AI field is often traced back to the year 2012. That year, Geoffrey Hinton’s research team demonstrated that deep neural networks could perform visual object recognition tasks. It’s well-known that different cortical areas of the brain correspond to different functions. Similarly, the various layers of deep neural networks are often analogized to brain regions such as V1, V2, and V4. However, the prefrontal cortex (PFC), situated just above the eyes, is still not sufficiently understood. Additionally, a functional equivalent of the PFC in contemporary machine systems has yet to be determined.
This enigmatic PFC region is crucial for various cognitive functions, including working memory and decision-making. Working memory refers to the brain’s capacity to maintain and manipulate information internally, thus enabling focus on inner thoughts without external distractions. Decision-making involves making choices under uncertainty.
In laboratories, working memory is typically studied using delay-dependent tasks. For example, a monkey is shown the location of food and then subjected to a delay phase of 5 or 10 seconds. During this delay, the monkey must remember whether the food was placed on the left or the right. After the delay, the monkey retrieves the food based on memory, not direct sensory stimuli (the food itself). In the early 1970s, using single-cell recording and electrical stimulation, it was discovered that individual PFC cells remained active during the delay phase. These neurons were not active before the stimulus and did not react to the stimulus itself but showed continued activity during the delay, which intensified as the delay period doubled. This phenomenon is known as ‘stimulus-selective persistent activity’.
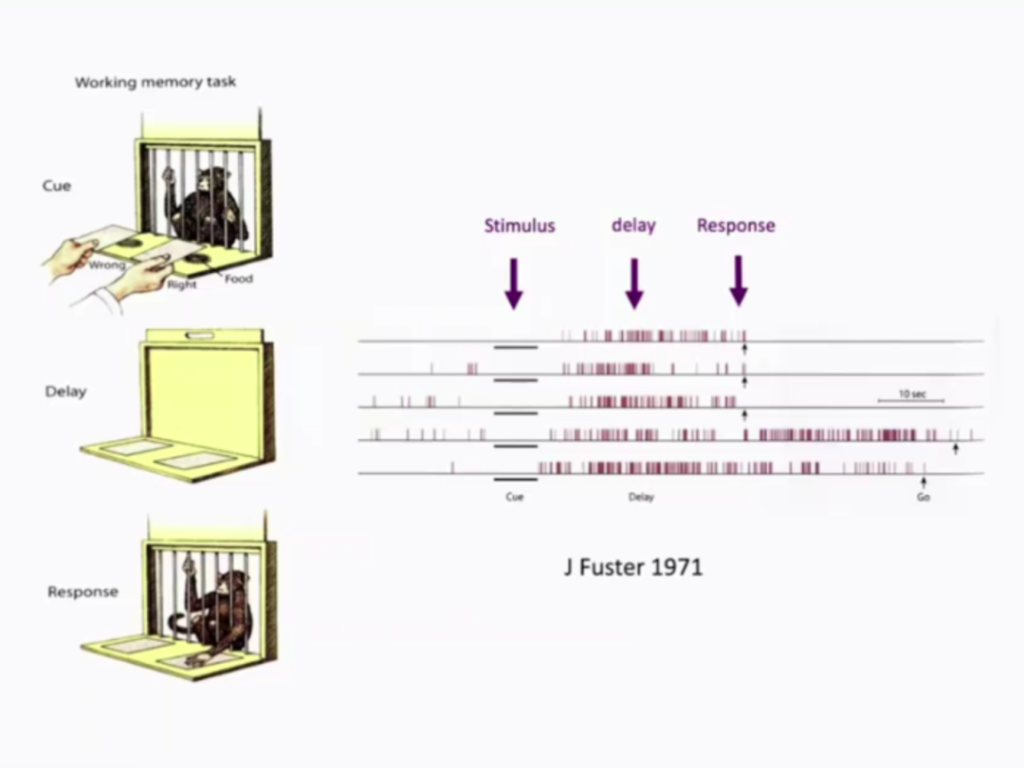
Caption: Delay-Dependent Task. Figure courtesy of Professor Xiao-Jing Wang.
In the study of decision-making, a simple yet ingenious experiment stands out: the two-alternative forced choice. Monkeys are trained to fixate on a point on a screen, and upon its disappearance, they make a decision: determining whether the movement of the point was to the left or to the right. Their decision is expressed through eye movements. The trick lies in the fact that, during any given trial, the proportion of the point’s movement direction, known as coherence or motion strength, is controllable. If all points move in one direction, the decision is easy. But if only 50% move in the same direction and the rest move randomly, it becomes more challenging. And with a mere 5% coherence, or even 0%, making a subjective judgment becomes exceedingly difficult. Single-cell physiological studies have shown that neurons exhibit ramping activity within milliseconds. This instantaneous and intense activity represents a mechanism through which neurons integrate information, accumulating evidence about different choice options to form a subjective judgment.
Professor Xiao-Jing Wang, through these two experimental examples, strikes at the core of the most complex issues. As the renowned psychologist Donald Hebb once said, ‘If one were to explain the delay between stimulus and response with a central neural mechanism, it seems remarkably congruent with the characteristics of thinking.’ When we think, it’s not driven by direct external stimuli but is an internal brain activity. Delayed activity is a specific instance of internal neuronal group activity.
However, the biological basis of decision-making or choice remains unclear, and AI systems lack this capability. As Noam Chomsky notes, ‘Once you get to issues of will or decision or reason or choice of action, human science is at a loss.’
Modeling Based on Biology
Despite the complexity of real biological processes, relatively simple behavioral paradigms can be used to attempt understanding mechanisms across different levels.
In a physics experiment involving a pendulum, if there is friction in the air, the pendulum’s amplitude decreases over time, exhibiting an exponential decay. Similarly, neuronal firing also decays over time, with a time constant of approximately 10 milliseconds. To achieve sustained activity in working memory, this issue needs to be addressed. Professor Wang proposes a bold hypothesis: reverberation. That is, if a group of neurons stimulates each other, they can continue to be active even after the input disappears. The strong horizontal connections in layers two and three of the PFC in primates provide a structural basis for this hypothesis.
To test this hypothesis, Professor Wang simplified the complexity by creating a system with only two dynamic variables. This system demonstrates how to integrate random dots, perceptual decision-making, and working memory for judgments and choices.
This model has two selective firing neuronal groups – a and b. There is reverberation within each neuronal group, but the excitatory selectivity between the two groups is much weaker. They compete through inhibitory neurons, ultimately resulting in a winner. This outcome represents the system’s choice. The system’s choice is determined by the motion strength and relative input to groups a and b. When the input disappears, the choice signal remains self-sustained throughout the delay period. This model can explain the basic processes of working memory and decision-making.
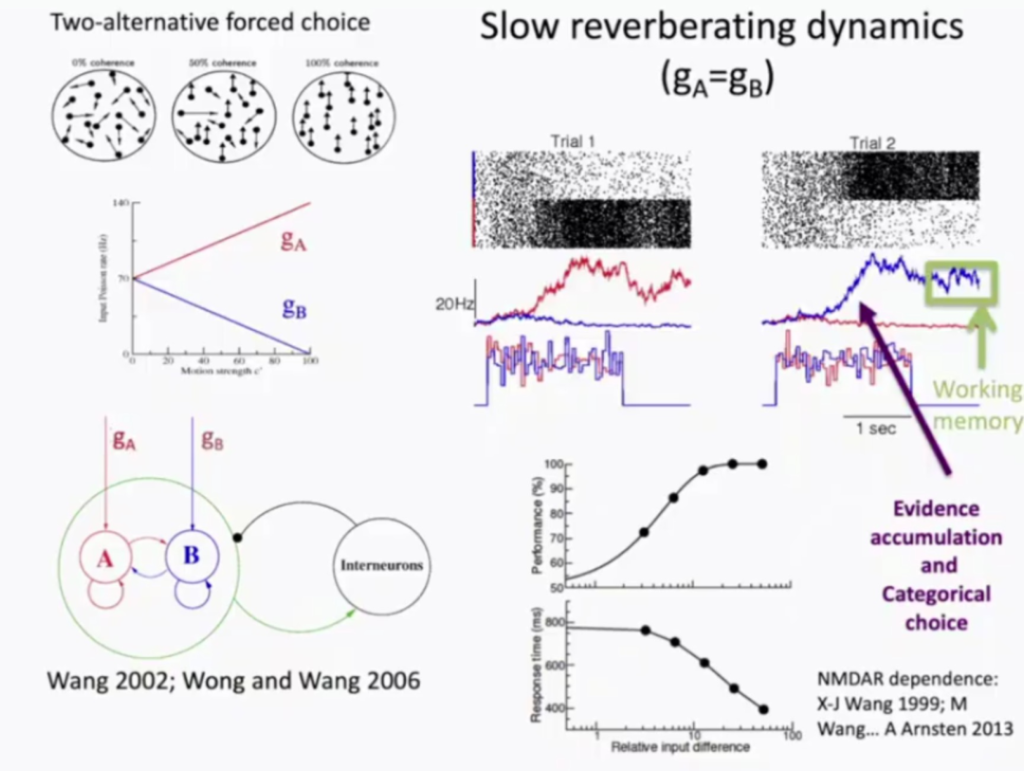
Caption: (Left) Two-Alternative Forced Choice; (Right) Slow Reverberation Dynamics System. Figure courtesy of Professor Xiao-Jing Wang.
Furthermore, this model operates across multiple levels. By considering reaction time as a function of motion strength, it is observed that the decision-making time increases as the motion strength decreases and the task becomes more difficult. This mirrors our everyday experience, where making a tough choice often requires considerable time to weigh different options. This phenomenon is also reflected in monkey experiments, elucidating why the dynamics of recurrent neural circuits can explain behavior.
Additionally, Professor Wang offers insights from a cellular and molecular perspective. For instance, slow time integration is primarily achieved through excitatory reverberation mediated by NMDA receptors. NMDA receptors are crucial for decision-making and working memory, as tested and confirmed in monkey experiments. If a drug were found that reduces or eliminates NMDA signaling, then neuronal activity would cease.
When considering self-sustained and internal states as functions of recurrent activation strength, and labeling these states with a parameter, it is found that in this model, above a certain parameter threshold, a series of stimulus-selective, persistent states emerge. These states include different memory items in working memory. The emergence of these states can be explained as bifurcations in the dynamic system, where graded changes in parameters lead to different functions and capabilities. This perspective is vital for recurrent neural circuits and is also applicable in multi-regional, large-scale brain systems.
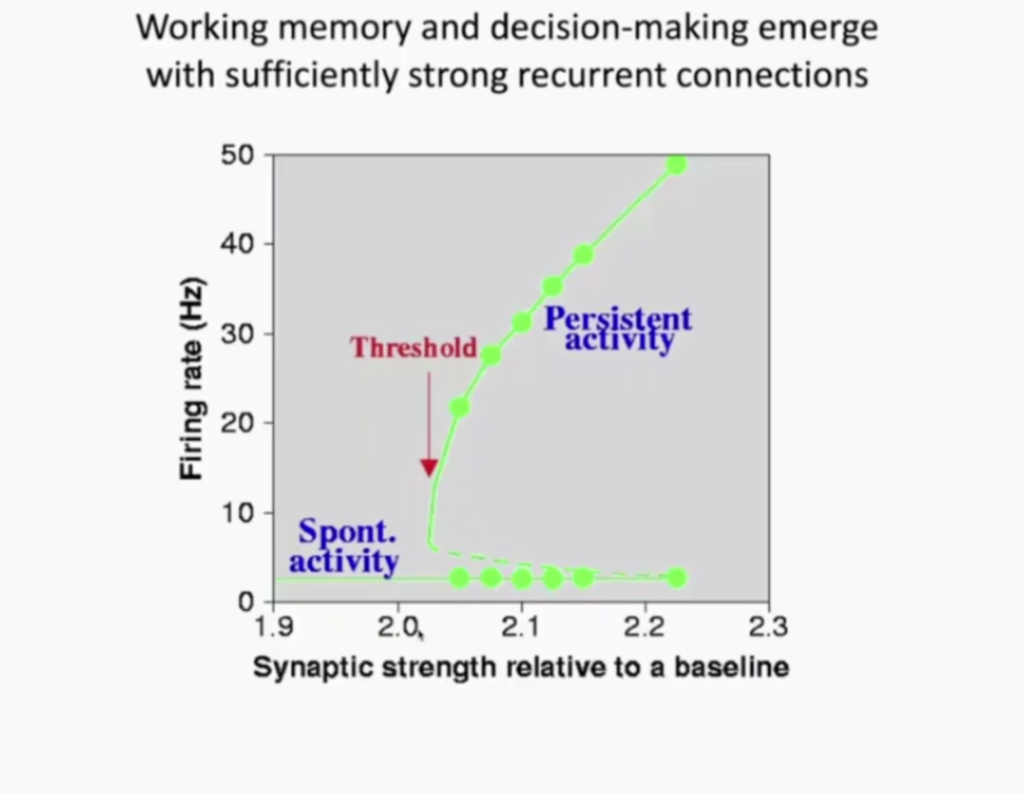
Caption: Sufficiently Strong Recurrent Connections in Working Memory and Decision-Making. Figure courtesy of Professor Xiao-Jing Wang.
Building upon this foundation, we can utilize machine learning to establish neural circuits akin to biological ones, capable of performing 20 different tasks, including working memory, decision-making, categorization, multisensory integration, delay sampling, delay categorization, and more. Once the network successfully executes these nuanced tasks, it enables us to ‘open the black box’ for analysis, pose questions, and delve into the dynamics of new circuits and neuronal groups. Neuronal activity can be gauged through task differentiation, and the interactions and organizational patterns among neurons can be deciphered using cluster analysis.
However, even if we design circuits that resemble the PFC, capable of flexibly guiding behavior based on rules, our endeavor should not end there. In reality, any given function involves multiple brain regions, including the PFC, the posterior parietal cortex, and potentially even supra-cortical structures.
From Local Networks to Brain Systems
The interactions between different regions of the brain exhibit a high degree of recursion. As a theoretician, Professor Wang asserts that merely qualitatively sketching their feedback connections is insufficient; he advocates for the clarity and precision of numerical descriptions. Over the past decade, technological advancements have made it feasible to utilize collected data for constructing directed and weighted connection matrices between various regions.
In the cortex of macaque monkeys, when the directed connection weights between two regions are represented in histograms, it is observed that these histograms across multiple regions follow a log-normal distribution. The weight of any pair of regions and their wiring distance exhibit an exponential distribution. This implies that traditional graphical representations of cortical networks fall short, as they are based on topological logic and do not incorporate this specific embedding, failing to reflect the unique relationships between cortical areas. Professor Wang’s team has developed a new class of mathematical models, specifically designed for this special embedding, enabling the study of real cortical networks.
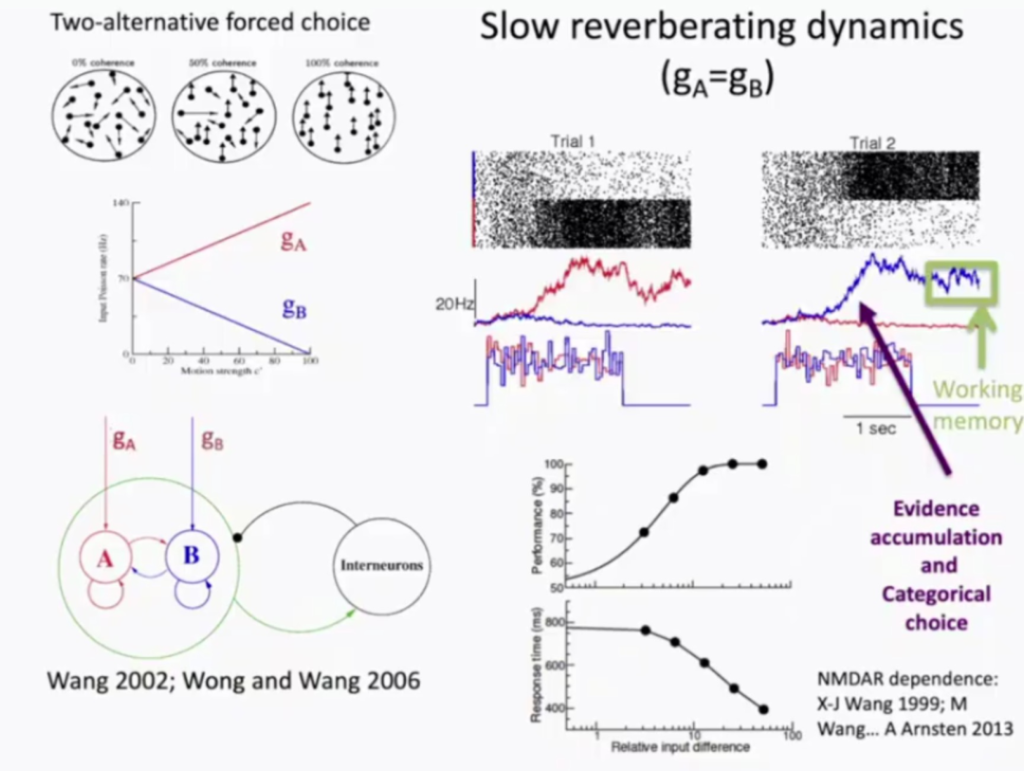
Caption: (Left) Graphical Representation; (Right) Histograms of Directed Connection Weights Between Different Regions and Histograms of Weight Against Wiring Distance. Figure courtesy of Professor Xiao-Jing Wang.
In constructing large-scale cortical models, mathematical modeling of each region is required. Different regions may necessitate distinct mathematical models. Therefore, the process begins by formulating scientific questions and establishing corresponding models based on these inquiries.
In neuroscience, the cortex column is a recognized principle, referring to a columnar structure composed of vertically aligned neurons with similar functional and structural characteristics. This represents a local circuit unit that is repeated many times, consistent across rodents, monkeys, and humans. However, even within the same local circuit, quantitative differences exist that lead to qualitative changes, namely, differences in function and capabilities. Thus, it’s essential to consider these quantitative variations in model construction.
Professor Wang’s research has demonstrated the impact of quantitative differences in large-scale systems. By analyzing dendritic spine numbers as a function of layer position, a systematic increase in the number of spines per cell is observed. This microscopic gradient reveals the relationship between the excitation intensity of neurons in different areas. In a recent paper published in Nature Neuroscience, Professor Wang studied the expression gradients of neurotransmitter receptors in the macaque cortex, such as dopamine and serotonin receptors. He discovered a cortical hierarchy in which receptors in higher-level cortical areas have higher density, larger dendrites, and fewer myelin sheaths. This is another example of using mathematical models to quantify changes in biological characteristics.
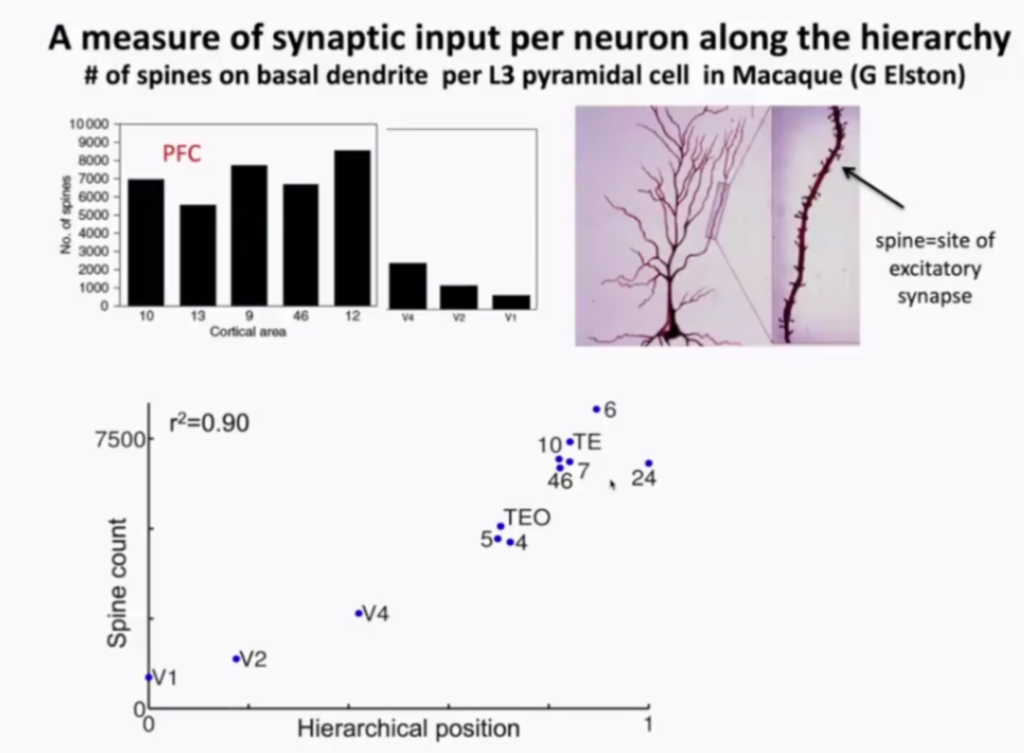
Caption: A Method for Measuring Neuronal Synaptic Inputs Along the Hierarchical Levels of Basal Dendritic Spines of Pyramidal Cells. Figure courtesy of Professor Xiao-Jing Wang.
Beyond the quantitative hierarchy, a hierarchy in time constants also exists. The temporal scales of activity in different regions vary, with some regions having shorter time constants and others longer. For instance, the visual network operates very rapidly. When watching a movie, the sensory input is constantly changing, necessitating a very short time constant for swift reactions. Conversely, in decision-making, neuronal activity incrementally increases, implying the need for a much longer time constant. This has significant implications for cognitive processes such as working memory and decision-making.
Professor Wang also notes that analyzing isolated regions reveals that connections between regions are not only local but also long-distance, forming a bifurcation space. Constructing large-scale cortical models involves both adding linear gradients and analyzing time constants. The hierarchical structure and time constants aid in understanding the impact on large-scale dynamics and function.
Finally, Professor Wang emphasizes that neural circuits are not all alike. Cognitive circuits like those in the PFC, capable of handling both working memory and decision-making simultaneously, present the most thought-provoking issues from an AI perspective.
In Conclusion
Neuroscience, being a complex and fascinating field, is enriched by numerous examples from computational neuroscience in this keynote address. It delves deep into the essence, uncovering patterns and trends hidden in the data and progressively revealing the workings of neural networks. Professor Wang, with his elegant physics and rigorous mathematics, narrates the process of conducting detailed biological research. The content, both profound and accessible, provokes deep reflection.